AI bias remains one of the most challenging problems in modern technology development. As algorithms increasingly shape our world, the question of whether we can ever build truly unbiased algorithms becomes critical. AI systems that make biased decisions can perpetuate or even amplify existing social inequalities across healthcare, finance, criminal justice, and employment. Moreover, these AI bias issues often emerge subtly, making them difficult to detect until after harm occurs. Despite significant advances in artificial intelligence, creating completely unbiased algorithms continues to elude even the most sophisticated development teams. Therefore, addressing AI bias requires not just technical solutions but also ethical frameworks and diverse perspectives throughout the development process.
The Root Causes of AI Bias
AI bias doesn’t materialize from nowhere—it stems from several identifiable sources. First and foremost, biased training data directly leads to biased algorithms. When AI systems learn from historical data that contains societal prejudices, they inevitably reproduce these patterns in their outputs.
For example, resume screening algorithms trained on hiring decisions from the past decade might inadvertently penalize women applicants if the historical data reflects gender discrimination in hiring practices. Similarly, facial recognition systems have consistently shown higher error rates for women and people with darker skin tones because their training datasets predominantly featured light-skinned male faces.
Additionally, bias can emerge from how developers frame problems. The choice of variables, definitions of success, and optimization targets all contain implicit value judgments that shape algorithm behavior. For instance, a lending algorithm designed to maximize profit might systematically deny loans to certain demographic groups simply because historical data suggests higher risk—perpetuating historical inequities rather than addressing them.
Types of AI Bias
Understanding AI bias requires recognising its various forms:
Selection bias: occurs when training data doesn’t accurately represent the population the algorithm will serve. This commonly happens when data collection methods systematically exclude certain groups.
Measurement bias: emerges when the features chosen as proxies for success contain hidden prejudices. For example, using ZIP codes as a predictive factor might inadvertently introduce racial bias due to historical segregation patterns.
Confirmation bias: appears when systems are designed to confirm existing beliefs rather than challenge them. This often manifests in recommendation algorithms that create “filter bubbles” by showing users content that reinforces their existing viewpoints.
Historical bias: exists when past societal inequities become encoded in seemingly objective data. For instance, historical wage data reflects gender pay gaps, which means algorithms trained on such data might perpetuate those disparities.
The Technical Challenges of Debiasing
Creating unbiased algorithms faces numerous technical hurdles. First, defining “fairness” mathematically proves surprisingly complex—researchers have identified at least 21 different definitions of algorithmic fairness, many mutually incompatible.
Furthermore, removing one type of bias often inadvertently introduces another. For example, making an algorithm “blind” to protected characteristics like race might seem like a solution, but can actually increase bias when those characteristics correlate with other variables in the data.
Another challenge involves the trade-off between accuracy and fairness. Sometimes, the most mathematically accurate model contains bias. Developers must then decide how much predictive power they’re willing to sacrifice for fairness—a value judgment rather than a purely technical decision.
The complexity increases further with modern deep learning systems. Their “black box” nature makes identifying the source of bias extremely difficult, as these models operate through millions of parameters without transparent reasoning processes.
Progress in Addressing AI Bias
Despite these challenges, researchers and companies have developed promising approaches to reduce algorithmic bias. One method involves preprocessing training data to remove or counterbalance biased patterns before algorithms learn from them. For instance, researchers might reweight examples to ensure demographic parity.
Alternatively, in-processing techniques modify learning algorithms themselves to penalize biased patterns during training. Some approaches incorporate fairness constraints directly into optimization functions.
Post-processing methods adjust already-trained models to equalize error rates across different groups. This approach proves especially useful when retraining isn’t feasible.
Meanwhile, diverse development teams help spot potential biases that homogeneous groups might miss. When teams include people from various backgrounds, they bring different perspectives to problem framing and evaluation.
Explainable AI (XAI) techniques also aid bias detection by making algorithms more transparent. These methods help users understand how systems reach decisions, making it easier to identify problematic patterns.
Regulatory and Ethical Frameworks
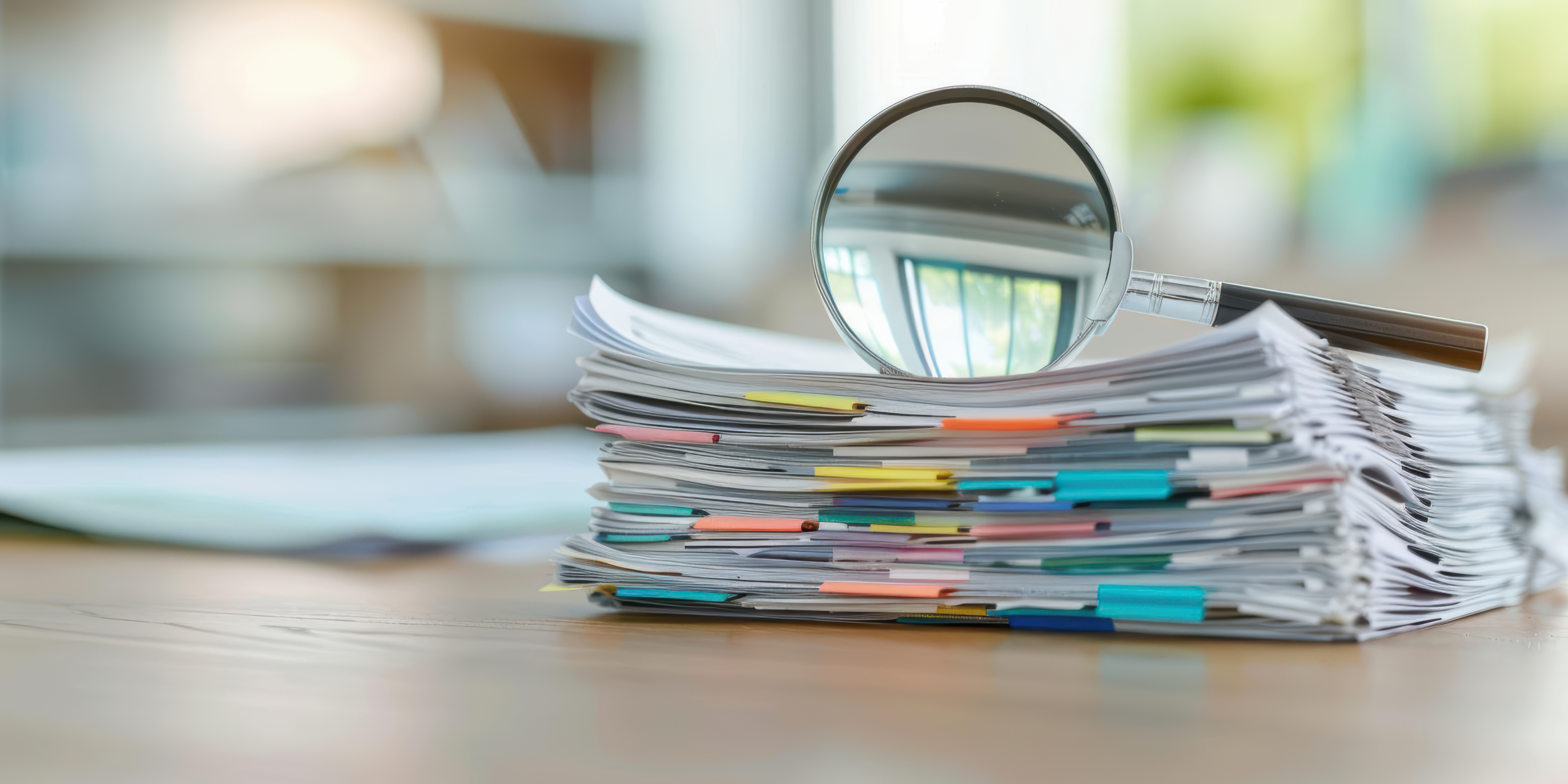
Technical solutions alone cannot solve the bias problem. Consequently, governments and organizations have begun developing regulatory frameworks to ensure algorithmic fairness.
The European Union’s AI Act explicitly addresses algorithmic discrimination, requiring companies to evaluate high-risk AI systems for bias before deployment. Similarly, in the United States, several state and local governments have passed algorithmic accountability laws.
Industry standards are emerging too. Organizations like the IEEE have developed ethical guidelines for AI development, while many companies now publish regular AI impact assessments.
These frameworks typically emphasize transparency, accountability, and meaningful human oversight. They recognize that even if perfect neutrality remains unattainable, responsible AI development requires continuous monitoring and improvement.
The Human Factor
Ultimately, algorithms reflect human values and choices. Therefore, addressing AI bias requires asking foundational questions about what kind of society we want technology to help create.
This means involving diverse stakeholders in AI governance—not just technical experts but also affected communities, ethicists, social scientists, and policymakers. Through inclusive deliberation, societies can decide which trade-offs between different definitions of fairness best align with their values.
It also requires acknowledging that neutrality itself represents a value position. Algorithms designed to maintain the status quo aren’t truly “neutral” if existing conditions contain structural inequalities.
Conclusion
Can we ever build truly unbiased algorithms? The answer appears increasingly clear: probably not in any absolute sense. However, this doesn’t mean we should abandon the pursuit of fairness in AI.
Instead, we should recognize bias as a multidimensional challenge requiring ongoing attention rather than a problem with a one-time solution. By combining technical innovations, diverse development teams, transparent processes, and robust oversight mechanisms, we can create AI systems that reflect our highest values rather than our historical mistakes.
The goal shifts from achieving perfect neutrality to building responsibly biased systems—algorithms whose inevitable value judgments are explicit, democratically determined, and aligned with principles of equity and justice. Through this approach, AI can become a powerful tool for creating a more fair and inclusive society rather than reinforcing existing inequities.
FAQs:
1. Why can’t we just remove race and gender from datasets to eliminate bias?
Simply removing protected characteristics doesn’t solve bias because other variables, like ZIP codes, can act as proxies. In some cases, explicitly including these traits helps detect and mitigate bias rather than ignoring systemic disparities.
2. How can companies test for AI bias?
Companies should test AI across diverse demographic groups, auditing error rates and outcomes. Bias detection tools, impact assessments, and user feedback mechanisms help identify and address unfair patterns.
3. Who is responsible for biased AI decisions?
The responsibility falls on organizations deploying AI, not just the algorithm. Developers, data providers, and regulators all share accountability, requiring oversight, monitoring, and ethical safeguards.
4. Can explainable AI reduce bias?
Explainable AI helps by revealing decision-making patterns, making bias easier to detect. However, transparency alone isn’t enough—active intervention is needed to correct unfair outcomes.
5. How does AI bias vary by application?
Bias manifests differently across fields—facial recognition struggles with demographic accuracy, hiring tools may filter out certain candidates, and NLP can reinforce stereotypes. Each requires tailored mitigation strategies.
6. How does data diversity impact AI bias?
More diverse data improves fairness but doesn’t eliminate bias entirely. If historical data contains discrimination, AI will still reflect those patterns, requiring additional bias-mitigation techniques.
7. Is algorithmic bias inevitable?
Some bias is unavoidable, as AI simplifies complex realities. However, harmful biases that reinforce discrimination can and should be minimized through transparency, ethical design, and ongoing oversight.
Stay updated with our latest articles on fxis.ai